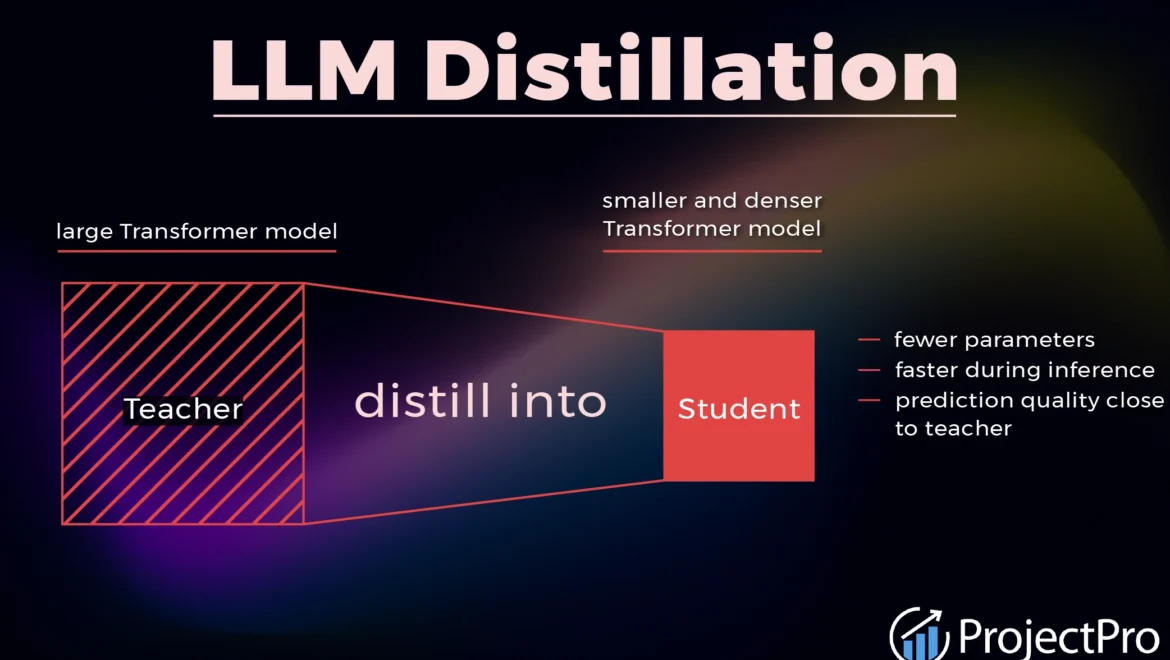
LLM Distillation is now new technique revolutionalising the way we are all going to use Generative AI and it is going to transform the agenetic AI world from the perspective of total cost of ownership for enterprises in implementing generative AI and embracing the value of Automation with AI. Recent innovative product DeepSeek-R1 actually is finest implementation of LLM distillation which has changed the perception of AI is costly.
What is LLM Distillation ?
It refers to the process of transferring the knowledge from larger complex model refer as Teacher model to a smaller and efficient model refer as Student model. The process of Knowldge distillation was first introduced by Geoffrey Hinton, attaching paper here. It was written in 2015. The goal of Distillation is to maintain the smaller model performance closer to teacher model by significantly reducing the computational resources for inference and deployment. Training smaller student model using the knowledge of teacher model. First teacher model generates soft labels. Soft labels are the right answers with probability as a result of knowledge it has from previous training iterations. These soft labels will help the student models with confidence levels rather than right or wrong answers. In addition to soft labels, student model also learns from ground truths. Both of these help student model learn faster reasoning patterns than memorising the answers. One the student model learns from teacher model it can be further fine tunes in task specifics and real world reasoning. These are smaller LLMs require smaller computational resources. They are efficient, cost savants are more and easy to scale.
There are challenges in Distilled models also. Loss of information and faster ensuring of generalization. It may not capture all nuances of larger teacher models.
Distilled models are better suited for Mobile devices, edge devices, low-latency and real time systems. For example DistillBERT is 40% smaller and 60% faster than BERT, which is Original Teachers model. Another example is DistillGPT-2, 40% smaller and 60% faster than GPT-2. It enables real time AI and less resource consumption.
0 comments
Write a comment